What Do We Mean When We Say: Language Model
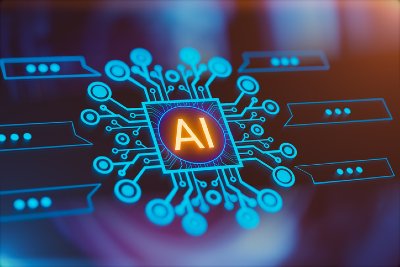
Artificial intelligence, more commonly referred to as simply AI, is one of the great emerging technologies of this computing generation. As with all things, AI didn’t reach IT, “stardom” overnight. Behind the seemingly meteoric rise of AI were years of hard work, innovation (and imagination), massive amounts of data/datasets, tests and retests led by IT scientists who had the vision to build upon existing technologies and use them in exciting new use cases.
It might be said that AI grew out of the roots laid down in language modeling. Without language modeling, in all likelihood there would be no AI today. Here, we’ll take a quick cruise around the world of language modeling and discovery why it’s so important.
What are language models?
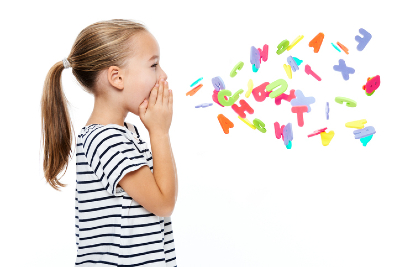
When it comes to learning languages, humans are naturally wired to learn to communicate. From infancy, we’re exposed to thousands of datasets in the form of words and gestures as we interact with parents, family, adults, other children, and our environment. At a high level, understanding language comes through listening to large amounts of speech “data.”
This eventually leads to word recognition, speech and finally, of course, mastery of the written language skills. As we mature, our language skill and understanding increases leading to the development of more complex communication skills.
Language Modeling (LM) is a word/language predictive tool. LMs possess the capability to “learn” human language. Instead of listening to large datasets of spoken words, LMs are “fed” large volumes of text datasets which are run through an algorithm and analyzed to establish a baseline for word predictions.
Using various probabilistic and statistical techniques, LMs are able to identify the likelihood or probability that a certain word or sequences of words will appear within the referenced text. This allows LMs to incorporate information in a reusable manner that is reflective of written human communication. Through this process, LMs learn to predict words or sentences, produce new text, and understand new content.
LMs and Artificial Intelligence
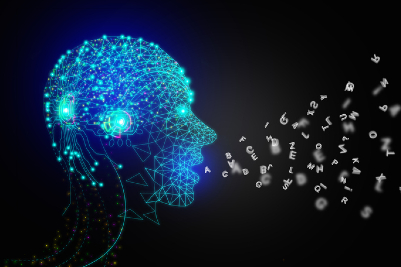
Artificial Intelligence (AI) (as opposed to human intelligence) is the science of developing “intelligent” machines that are able to perform tasks traditionally performed by humans and that require human intelligence. This may include tasks such as real-time translation between different language or speech recognition.
LM systems are an essential component of AI. Natural LM systems leveraged by AI include:
Natural Language Processing (NLP): Used to enable computers to understand written and spoken speech in real time.
Natural Language Understanding (NLU): Analyzes language; understands sentences not just words.
Natural Language Generation (NLG): Used in human-machine interactions; Able to produce spoken and written narratives from datasets.
Use cases for LMs are plentiful and chances are that most of us have been using AI technology built upon LMs for quite some time. Have you ever uttered the words “Hey, Siri” or “Alexa?” Perhaps you love the hands-free calling feature that comes factory standard in numerous vehicles where making a call is as easy as the simple command, “Call Dad.” All of these are examples of speech recognition, an NLP use case.

Machine translation is another popular use case that employs LM. Machine translation involves the translation of one language into another in real time. For those studying a new language, applications such as Google Translate can be your best friend!
The practical application of machine translation extends far beyond simply looking up words and phrases. A child services case manager recently shared with me that one of her clients speaks no English. The case manager regularly uses the Google translate feature to communicate with her client.
Many email providers or grammar applications actively “suggest” or “recommend” text for sentence endings based on the text already entered. Of course, as a writer, I prefer my own wit and words to those suggested by the wisdom of AI!
Other common use LM-based used cases include features such as text generation, chatbots, optical character recognition (used to digitize records), parsing, parts-of-speech tagging, information retrieval, and emotion (sentiment) analysis in writing.
To infinity and beyond!
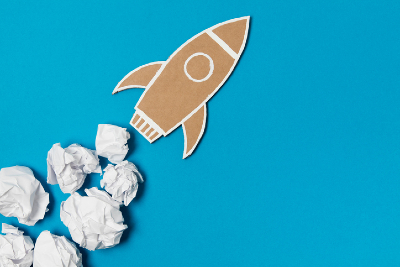
Language Modeling, is the foundation of AI. Different types of LMs are suited to different types of tasks. Regardless of the type of LM use, language modeling is the technology bedrock that enables machines to understand information that is qualitative in nature, analyze the information and then turn qualitative information into quantitative information.
Qualitative information is measurable and numbers-based. This type of data provides information such as how much or how many. On the other hand, qualitative information is descriptive and interpretive. Qualitative data explains behavior by addressing not only what happened but how and why an action occurred. Without LMs, humans would not be able to “communicate” with machines in the same manner which is used today.
LM technology is an equal opportunity technology which is actively used across most industry sectors. As LMs become more complex and continue to evolve, the AI systems that rely on LMs will continue to evolve as well leading to new and emerging use cases and expansion into new industry domains.
One interesting innovation is the adoption and use of pre-trained language models. Pre-trained language models eliminate the need for enterprises to develop LMs from the ground up. Using pre-trained language models reduces the need for large training datasets, provides adaptability and flexibility, and reduces the time required to implement and obtain results.
It’s likely that pre-trained datasets will reduce costs associated with the adoption of AI technologies making it more accessible and affordable for small- and mid-sized businesses to expand into the AI world.
Applications such as ChatGPT and Bard are two rising stars which have captured global attention. Both applications possess the ability to understand complex speech and language patterns along with the ability to produce very humanlike text. In 1972, Memorex advertised the quality of their cassette tapes with an ad campaign featuring the iconic line “Is it real, or is it Memorex?” In the future, we may well be asking “Is it real (i.e. human created) or is it AI?”
Footnote: Cautionary Concerns

Because the applications of LM-driven AI are limited only by the imagination, future usage is not without some concerns. Two common concerns include ethical considerations and socio-economic impacts to consumers and the labor force.
1) Socio-Economic Concerns — Currently, the development and use of AI remains largely unregulated leaving opportunities for technology abuse. According to the Centre for Economic Policy Research (CEPR) rather than providing opportunities for the labor force, unregulated AI may actually be detrimental and lead to adverse outcomes for the labor force and market.
In particular, CEPR cites loss of job, inequality in product and labor markets, consumer welfare and price competition, consumer behavior manipulation, and wage suppression as some examples of negative social-economic impacts. Additional social impacts include the effect of AI on news consumption, politics, democracy, surveillance and repression applications, and social communications.
2) Ethical Concerns — Potential bias in an ongoing dilemma in LMs and AI. Datasets frequently contain information based on local customs and culture, and stereotypes. If datasets contain misinformation, the results are not neutral, causing results to be biased. Common bias may include gender, religious, political, or nationality. which may influence the generated results. Depending on the algorithms used, search engine results may be biased depending on consumer usage, locality or other specific factors.